Gaussian Mixture Model Sine Curve¶
This example highlights the advantages of the Dirichlet Process: complexity control and dealing with sparse data. The dataset is formed by 100 points loosely spaced following a noisy sine curve. The fit by the GMM class, using the expectation-maximization algorithm to fit a mixture of 10 gaussian components, finds too-small components and very little structure. The fits by the dirichlet process, however, show that the model can either learn a global structure for the data (small alpha) or easily interpolate to finding relevant local structure (large alpha), never falling into the problems shown by the GMM class.
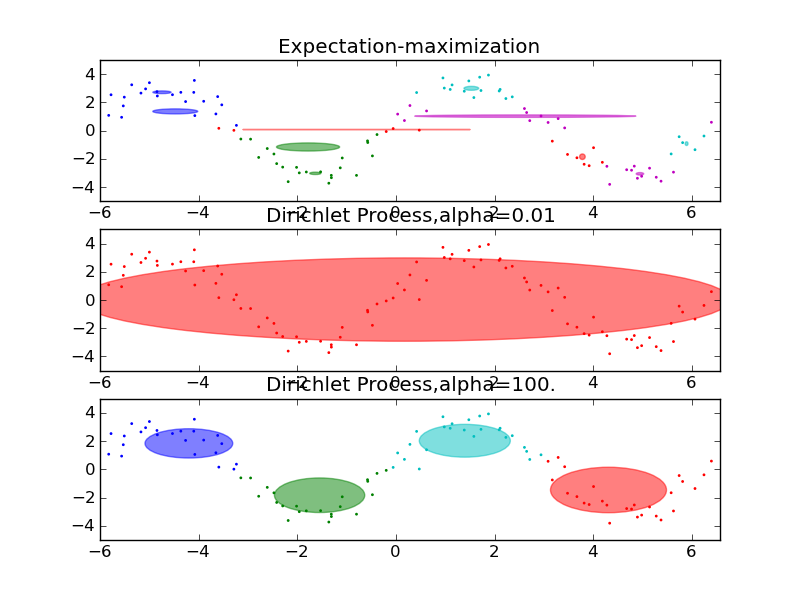
Python source code: plot_gmm_sin.py
import itertools
import numpy as np
from scipy import linalg
import pylab as pl
import matplotlib as mpl
from sklearn import mixture
# Number of samples per component
n_samples = 100
# Generate random sample following a sine curve
np.random.seed(0)
X = np.zeros((n_samples, 2))
step = 4 * np.pi / n_samples
for i in xrange(X.shape[0]):
x = i * step - 6
X[i, 0] = x + np.random.normal(0, 0.1)
X[i, 1] = 3 * (np.sin(x) + np.random.normal(0, .2))
color_iter = itertools.cycle(['r', 'g', 'b', 'c', 'm'])
for i, (clf, title) in enumerate([
(mixture.GMM(n_components=10, cvtype='diag'),
"Expectation-maximization"),
(mixture.DPGMM(n_components=10, cvtype='diag', alpha=0.01),
"Dirichlet Process,alpha=0.01"),
(mixture.DPGMM(n_components=10, cvtype='diag', alpha=100.),
"Dirichlet Process,alpha=100.")
]):
clf.fit(X, n_iter=100)
splot = pl.subplot(3, 1, 1 + i)
Y_ = clf.predict(X)
for i, (mean, covar, color) in enumerate(zip(clf.means, clf.covars,
color_iter)):
v, w = linalg.eigh(covar)
u = w[0] / linalg.norm(w[0])
# as the DP will not use every component it has access to
# unless it needs it, we shouldn't plot the redundant
# components.
if not np.any(Y_ == i):
continue
pl.scatter(X[Y_ == i, 0], X[Y_ == i, 1], .8, color=color)
# Plot an ellipse to show the Gaussian component
angle = np.arctan(u[1] / u[0])
angle = 180 * angle / np.pi # convert to degrees
ell = mpl.patches.Ellipse(mean, v[0], v[1], 180 + angle, color=color)
ell.set_clip_box(splot.bbox)
ell.set_alpha(0.5)
splot.add_artist(ell)
pl.xlim(-6, 4 * np.pi - 6)
pl.ylim(-5, 5)
pl.title(title)
pl.show()